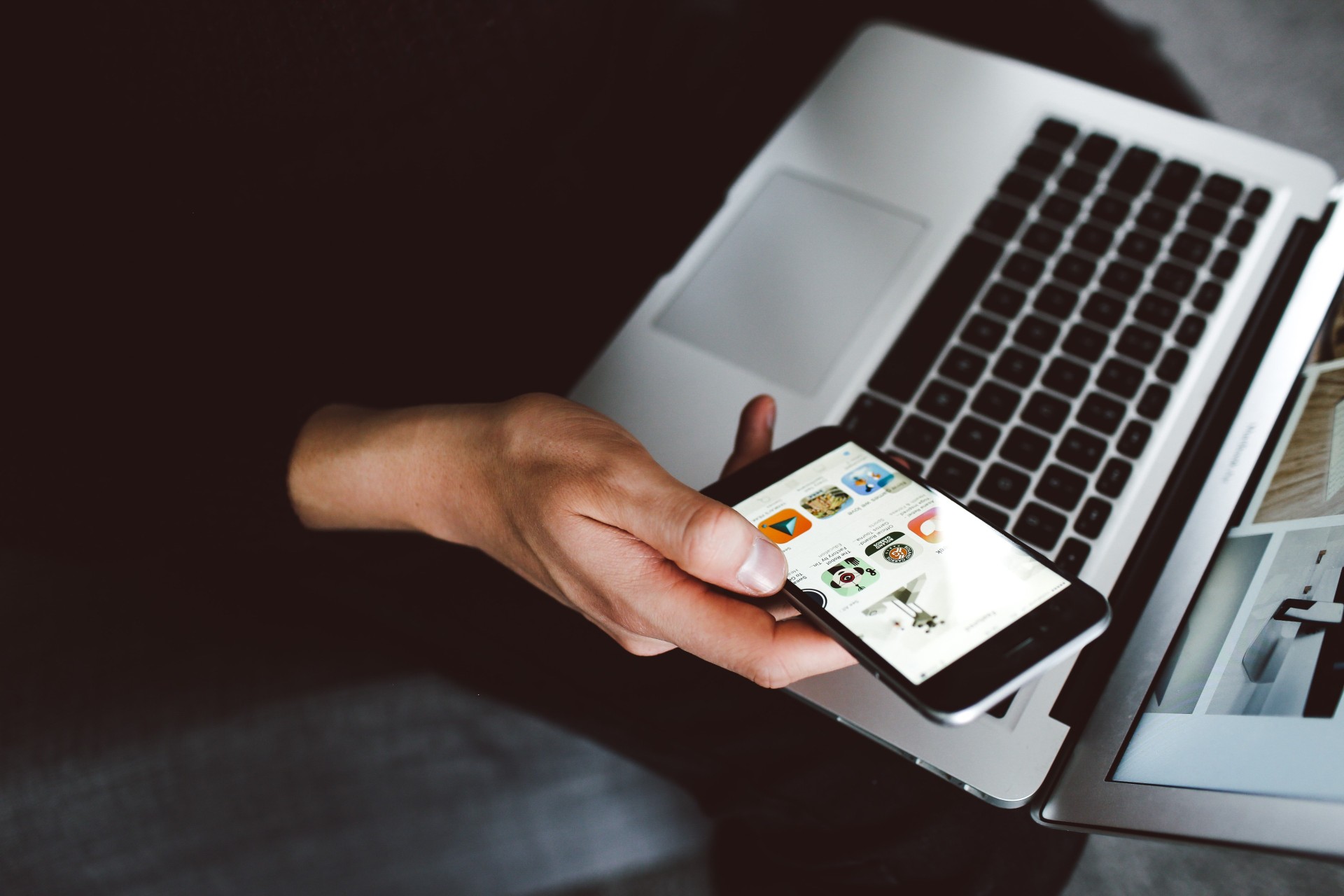
Everything You Need to Know About Attribution Modelling
How do you know if you are getting value from digital marketing?
It’s a question that drives many marketing professionals and business owners to distraction, not least when you have a limited budget and need to get the most out of it.
The difficulty is, there are just so many ways to reach and influence people online these days. Online advertising, SEO, social media campaigns, email marketing, the content on your own website. It all has a role to play. But how do you get the best out of it all?
And then you have to factor in that there are an equally large number of ways and places – we call them ‘channels’ in marketing speak – for customers to interact and (hopefully) buy from you.
Modern shopping ‘journeys’ – the sequence of events that people go through from pondering what to buy to actually making a purchase – are often complex and convoluted, taking in perhaps dozens of points of contact with your brand (or ‘touchpoints’) triggered by your marketing activity across a range of different channels.
It can be challenging enough just mapping out what kind of routes to purchase your customers take, especially as they will frequently hop back and forth across different channels, revisiting reviews and blogs and product pages etc in non-linear fashion, before making their final decision
With that kind of complexity, how do you start unpicking such a tricky notion as value and influence from all the different variables?
The solution most marketing professionals lean on these days is attribution modelling. Given the opaque nature of the challenge it tries to resolve, attribution modelling is itself one of the trickier areas of marketing to get your head around. Here’s a breakdown of the key things you need to know.
What is attribution modelling?
Attribution modelling is a form of data tracking and analysis. In a nutshell, it looks at all the different touchpoints available to customers across your business and seeks to analyse their contribution to the big prize you’re always striving for – transactions.
Because digital shopping journeys are often so complex and nonlinear, it’s rare to be able to say with any certainty that a customer made a particular purchase as a direct result of seeing an advertisement, or a recommendation on social media, or because they typed the product straight into a search engine and saw your ecommerce site top of the rankings.
More often than not, a customer will weave their way between multiple touchpoints across several channels before finally deciding to buy. Google’s marketing research blog Think With… has even suggested that consumers regularly go through anything between 20 and 500 touchpoints before deciding to buy. Yes, five hundred. How do you unpick which ones had the biggest influence on the decision to buy out of that?
The answer attribution modelling provides is to score and then rank them. By tracking everything a customer has done in the lead up to a purchase – the first principle of attribution modelling, if you like – you know which touchpoints have been used, you know what order they came in, you know which were repeated and so on.
By scoring and ranking them in terms of influence, you can then start to make comparisons across purchases. And that’s when you see which ones are having the biggest impact on the most sales.
How does it work?
The first thing you need for attribution modelling is data that shows all the steps a customer has taken to get to the point of purchase. In the modern era of web tracking, that’s pretty straightforward. Popular data tools like Google Analytics offer it as a matter of routine.
Not so straightforward is the scoring and ranking of the relative value (i.e. influence) of each touchpoint. An attribution model is the set of rules you create to do the scoring. There is no single universally accepted method, so attribution modelling always involves a choice between several competing approaches. Some of the most commonly used attribution models are as follows:
FIRST-TOUCH, LAST-TOUCH AND U-SHAPED ATTRIBUTION
The simplest attribution models, these are also considered the crudest and therefore of most limited use. First-touch attribution gives 100% credit for a sale to the first touchpoint in the journey, last-touch attribution gives 100% credit to the last. U-shaped attribution splits the difference between the two.
There is value in knowing if any channels or campaigns tend to initiate journeys that convert more than others, and the same applies to seeing if any seem to secure the decision to buy more often than most. But these approaches offer little if, say, you discover that conversions start and end in different places with similar frequencies. They also offer no insight into what happens in between.
MULTI-TOUCH ATTRIBUTION
Unlike first-touch, last-touch and U-shaped attribution, multi-touch models assign value to all the touchpoints in a sales journey. This lends itself to more nuanced insights. But there are different ways to go about it, with varying degrees of complexity and different benefits.
For example, linear attribution splits credit for all touchpoints in a sequence equally. So if a customer sees an ad then reads a review before deciding to buy, those two points of influence get 50% of the credit. If a customer ends up hitting 10 different touchpoints, it’s 10% each. This has some use for comparing how different channels and assets perform across all sales. But you have to be wary of bias against shorter journeys and how you account for customers going back to the same touchpoint more than once. And it fails to account at all for different weighting of influence within a single journey.
Another example of multi-touch attribution is called time-decay attribution. This does make an attempt at weighting the influence of all touchpoints in a single journey based on how close they came to the purchase. The last touch gets the highest score, and then the rating for each of the others declines gradually back to the first.
Some people like to flip the logic of this on its head and weight first touch most, with scores declining up to the last. Or, you could apply the same principle to a U-shaped distribution, with first and last touchpoints receiving the highest scores, and all the others dipping in value to meet at the lowest score in the middle.
Different versions of a time-decay model are useful in different situations. A classic model that weights the last touchpoint before conversion most heavily works well for longer purchasing journeys where tactical relationship-building and nurturing is important to securing a sale, as it conceptualises a careful step-by-step process. If your business tends to rely on shorter, less structured customer journeys, however, and especially if you find customers tend to start in lots of different places, a ‘reverse’ decay model can be most effective for revealing the most commonly taken (and therefore valuable) routes.
CUSTOM ATTRIBUTION
Finally, some marketing professionals don’t like the idea of fixed attribution models at all. They argue that every business is unique, with different blends of channels and different routes to purchase available to customers. Plus, you wouldn’t expect different companies to run identical marketing campaigns, would you?
To accurately understand what does and does not influence customers as they move ‘down the funnel’, to borrow from traditional marketing speak, you have to take these unique characteristics into account by building custom attribution models.
Custom attribution most commonly involves weighting touchpoints according to known intelligence about how important they are to the company (or even how important you want them to be), regardless of where they occur in a journey. This is great, but it requires data on attribute performance prior to creating the model, or adjusting models over time according to the picture they paint of how customers are behaving.
You can go as far down the rabbit hole as you want to (or are capable of) with this. You might, for example, want to understand how touchpoints influence one another, how certain sequences might increase or decrease the likelihood of conversion. With enough data you can start to draw these kinds of insights out from attribution models, with a view to then going back and shifting the weighting to reflect what you know about the most successful sequences. It’s not easy, although the advent of machine learning AI opens the door to attribution models automatically customising and refining themselves as they ‘learn’ from the patterns seen in previous data.
What can you achieve with attribution modelling
Traditional web metrics like traffic figures, linger time, unique visitors etc paint with broad brushstrokes a picture of how your digital channels are performing. You can be confident, for example, that if you have more people visiting your ecommerce site, you will probably end up with more conversions.
But what they don’t tell you is how you can actively influence more conversions. Attribution modelling can. By providing a model for scoring influence on eventual purchases across the complex mesh of touchpoints and channels you offer, it tells you what works and what doesn’t, which routes through the maze to transaction are efficient and effective, and which are little visited or end up in cul-de-sacs.
With that kind of insight, you can focus on laying down the most effective pathways that get people from the initial click through to buying as often as possible. You can also optimise the routes, ensuring customer journeys are consistent, convenient and quick by cutting out anything that doesn’t contribute, or that is even likely to confuse or hold up the customer.
With lean, purposeful, optimised journeys, you can be confident of increasing conversions and driving revenue. And you can also be happy that the marketing strategies you are pursuing are delivering value.
To find out more about attribution modelling, contact the Key Element data intelligence and analytics team.
SHARE: