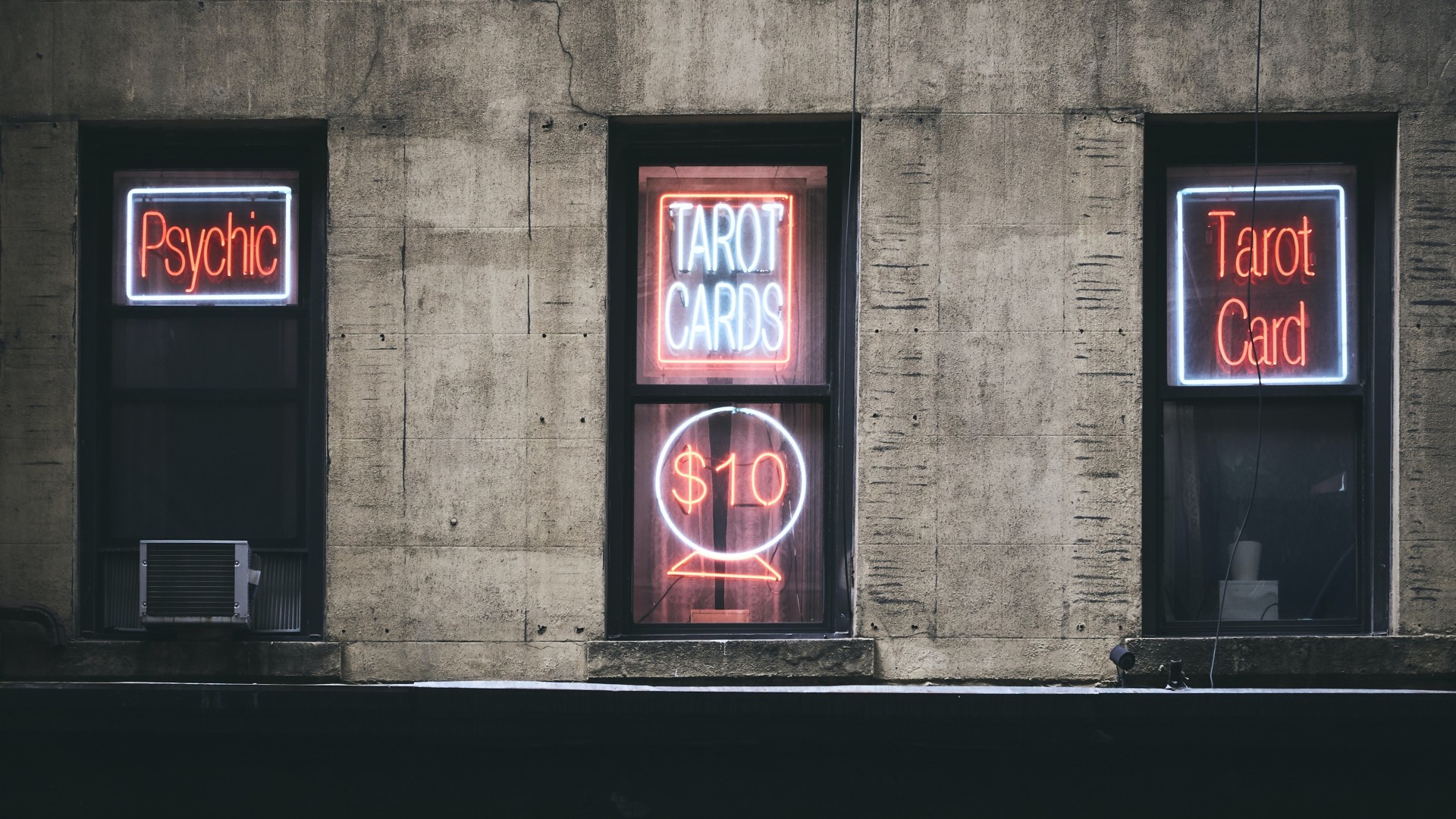
What is Predictive Analytics and Why Does It Matter To Your Business?
Every business owner would love a crystal ball to see the future with. The thin line between success and failure in business is often defined by how well companies anticipate and respond to future trends.
After all, markets are dynamic and ever-evolving. The firms that sense the winds of change and move quickly, those that spot ways to provide customers with better value, service and experiences ahead of their competitors are the businesses that forge ahead.
More prosaically, organisations that can forecast their own peaks and troughs in performance with a degree of accuracy that lets them smooth out the lows and maximise the highs will improve their outcomes considerably over time.
No one has yet invented a crystal ball that allows business owners to observe future market trends and their own performance ahead of time (although given the current rate of technological progress, don’t go ruling it out completely). But we do have the next best thing – predictive analytics.
What is predictive analytics?
Predictive analytics is a branch of data analytics. As its name suggests, it is concerned with using data to make predictions about what might happen in the future.
How does that work? There is no shortage of data available to modern businesses. As operations become increasingly digitised, as we do more and more online and let software take control, data is generated for every aspect of how a business functions. It’s one of the fundamental characteristics of digitised, computer-led systems – everything can be logged in bits and bytes.
But the data digital systems are so efficient at recording is always from processes that have already happened. Or, at very best, that are happening now. Data provides a record of what has happened, not what is going to happen.
Sophisticated in-depth analysis of processes and performance in the past tense is in itself very useful to businesses. It’s particularly powerful in the context of tackling issues and challenges, as it reveals hidden inefficiencies, bottlenecks and areas of waste.
So called ‘Big Data’ analytics – analytics at scale with massive data sets – has made a huge difference to business optimisation over the past decade. It has handed companies a previously unimaginable level of insight into how their businesses operate and perform. The more you know, the more you can effect positive change.
But still, this all essentially reactive. A case of analysing what has happened and applying a fix after the fact. Predictive analytics represents a move from reactive to proactive data-led decision-making. It doesn’t just seek to resolve or optimise what has already gone before. It seeks to anticipate future challenges and opportunities and empower businesses to act in advance.
How does it do this? Predictive analytics seeks to identify patterns in historical data and extrapolate future trends from them. It combines data analytics with statistical modelling, working in the realm of probability. Increasingly, it also incorporates Machine Learning – the area of AI where algorithms ‘learn’ from exposure to more and more data over time, using analysis of patterns to refine output.
In other words, AI-driven predictive analytics gets better and better at predicting the future the more data it has to work with, or the longer it runs for.
What can predictive analytics do for your business?
An obvious question that arises from this is – just how accurate is predictive analytics? If we’re talking about probabilities, rather than certainties, to what extent can any business rely on a forecast that comes from a piece of predictive analytics software?
The first point to make here is that, when it comes to the future, nothing is certain. So putting predictive analytics up against an imagined (and fantastical) ideal of 100% forecast accuracy misses the point. What we should be comparing it to is the methods traditionally used by people in business to forecast future trends – a blend of experienced intuition and educated guesswork.
Predictive analytics doesn’t need to be 100% accurate to be a better option than even the most skilled intuitive guesswork. By delving into datasets far too large for any person to realistically work with, identifying trends and patterns and applying statistical rigour to the probability forecasts derived from them, modern self-improving Machine Learning models can comfortably achieve accuracies of between 70 and 90%.
Just think about the impact that could have on your business – you nail between seven out of 10 and nine out of 10 of your future predictions. That really is the next best thing to a crystal ball.
So where is this predictive power best applied? There are many use cases for predictive analytics in business and the number is growing all the time. Here are just a few of them:
SALES AND MARKETING
Customer Relationship Management (CRM) software now routinely includes predictive algorithms that ‘lead score’ prospects and customers, or use individual and demographic behaviour data to tell you who is most likely to buy.
MANUFACTURING
Factory bosses can analyse equipment maintenance and failure records to inform service cycles. Known as predictive maintenance, the idea is to ‘fix’ machines before problems occur, and therefore boost up time. Predictive analytics is also widely used in manufacturing to support lean production, i.e. forecast fluctuations in demand to match output to it for optimum efficiency.
FINANCE AND INSURANCE
If you’ve ever applied for a loan or a credit card, you’ll have had to undergo a credit check. Credit checks and credit scoring nowadays use predictive analytics to give a more nuanced picture of risk. Similarly, insurers increasingly use predictive analytics to inform pricing decisions based on risk analysis.
ENERGY
One that is very topical right now, energy wholesale and retail providers use predictive analytics to set prices based on future forecasts of demand and availability.
RETAIL
Modern inventory management systems draw on sales data to forecast future demand for different products and ensure that stock and merchandising reflect what people want at different times. This can take into account things like seasonality, weather, new range availability etc.
Finally, especially with the growing influence of AI, predictive analytics lays the groundwork for what is known as prescriptive analytics. Predictive analytics provides probability-based forecasts to help inform decision-making. Prescriptive analytics takes the next step in applying rule-based techniques to recommend the best courses of action based on the predictive model, or even automate decision-making.
Interested in finding out more? Our data specialists offer expertise in a range of analytical techniques, predictive analytics included, that will help you cash in on your digital data. Contact us to learn how.
SHARE: